Ryan A. Metcalf, MD, CQA(ASQ), ARUP section chief of Transfusion Medicine, has partnered with machine-learning experts and clinicians at the University of Utah to develop and validate a machine-learning method that can predict the need for transfusion during cardiothoracic surgery. Their method predicts the likelihood that a patient will require large amounts of blood products. It can even predict how many red blood cell (RBC) units might be needed for patients with low risk.
Their research report, “Development and validation of a machine-learning method to predict intraoperative red blood cell transfusions in cardiothoracic surgery,” was published January 25 in the journal Scientific Reports.
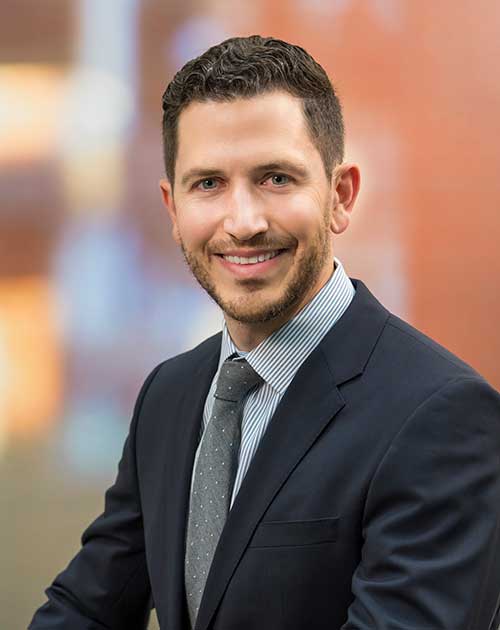
When Metcalf began the project in the summer of 2019, he recognized that existing available data could be used to predict important patient outcomes but were not being used to that data’s full potential.
“My first thought when starting this project was: How can we better use existing data to improve what we do every day in a tangible, meaningful way?” said Metcalf.
Cardiothoracic surgery will sometimes lead to massive hemorrhaging and can require massive transfusion more often than other types of surgery. Metcalf hopes that by providing a more accurate prediction of a patient’s risk of significant hemorrhage and need for transfusion in cardiothoracic surgery, this method will help optimize the use of blood products and prevent waste of a precious, limited resource, as well as help surgeons and anesthesiologists assess patient risk before surgery.
Currently, it’s standard practice for surgeons to order a specific amount of blood products before a specific surgical procedure; this amount is allocated to the patient in case of bleeding. Metcalf currently uses a data-driven maximum surgical blood order schedule (MSBOS), but all orders are set at 90th percentile of historical use for that procedure (a one-size-fits-all approach). MSBOSs are helpful but do not allow for personalized, accurate predictions. Reserving blood that is not transfused in some cases ties up RBC inventory that could otherwise be available for patients with unexpected massive hemorrhaging, such as in the case of trauma. It also increases the likelihood that any unused blood products may expire and be wasted.
The intermittent blood shortages caused by decreased blood donations during the COVID-19 pandemic have intensified the need to refine transfusion practices to ensure that blood products are available for patients in critical need.
More accurate machine-learning-based predictions that can identify patients at high risk for hemorrhage may also help surgeons employ mitigation strategies to prevent massive blood loss, as well as reduce the likelihood of overtransfusion, which carries its own risk of adverse effects.
“Transfusion is an activity that is performed frequently and can be lifesaving, but it is sometimes still overperformed,” Metcalf said. “Using data to better optimize how we approach transfusion medicine seemed like a natural progression.”
Building a Hybrid Machine-Learning Model
To develop their hybrid, machine-learning method, Metcalf and his colleagues used data from cardiothoracic surgeries that occurred at University of Utah Health from May 2014 through June 2019.
“To solve such a complex problem, we needed to smartly combine different approaches because no single method can accomplish what’s required. We leveraged different methods at each step, and then combined them in a pipeline-like procedure to get the final results,” said Shandian Zhe, PhD, an assistant professor in the U School of Computing who also worked on the project.
The members of the project first used an algorithm called Random Forest, which uses decision trees to categorize data, to limit the large number of variables available in the data to the factors that most correlate with risk of transfusion. The top 10 indicators included factors such as those related to the use of extracorporeal membrane oxygenation (ECMO), which involves a machine that helps circulate oxygenated blood throughout the body when the heart cannot, hemoglobin and calcium levels, and thoracoabdominal aortic aneurysm repair (a surgical treatment to repair an aneurysm in the aorta).
Once they identified the most significant factors, the team then used a Gaussian process to classify each case into one of two categories: low risk or high risk (the latter defined as cases that required four or more RBC units). For cases with low risk, they were also able to predict the number of RBC units those cases required with reasonable accuracy.
However, identifying the specific blood requirements for high-risk cases proved more difficult due to the smaller sample size, which was expected. Therefore, the final hybrid model first classifies cases as low risk (three or fewer RBCs transfused) or high risk (four or more RBCs transfused). The model is then used to predict the exact amount to be transfused only for cases initially classified as low risk.
Metcalf believes that other institutions could implement a similar method to create better predictions for how much blood patients will need for major surgery, and that this method will ultimately improve their blood management practices and conserve a vital resource.
“The approach itself may be more generalizable, rather than using the specific model, because there may be variation and nuances in how different institutions practice,” said Metcalf.
Is Data-Driven Transfusion Medicine the Future?
The next step in this project is to implement the knowledge gained from these prediction models in transfusion practice. Metcalf hopes that further research and development will eventually result in an application that will allow clinicians to access real-time data to inform clinical decisions.
“We envision an application that would be simple for the end user to view and interpret, as well as safe,” said Metcalf.
Not only can data predictions lead to better blood management practices, but the insights gleaned from the process, such as identification of the most significant predictive factors, could lead to a better understanding of what causes hemorrhage and better prevention strategies in the future.
While using data predictions to inform transfusion practices has the potential to improve care and even save lives, there are also challenges to implementing this knowledge, especially in medicine.
“Data access and management are critical issues in medical applications,” Zhe said. “To build functional applications, we need access to real-time data, but we also need to ensure that data are anonymous to protect patient privacy.”
Nonetheless, this research represents an important step forward in understanding how machine-learning models might be used to improve medical practice.
Metcalf is also working on other ways to apply machine learning to transfusion medicine, including a model that can accurately predict which patients are at risk of in-hospital mortality.
“This could be a big part of the future of transfusion medicine: learning how to apply machine-learning approaches as safely and as effectively as possible as we work toward a more data-driven, transformative practice,” he said.
Kellie Carrigan, kellie.carrigan@aruplab.com